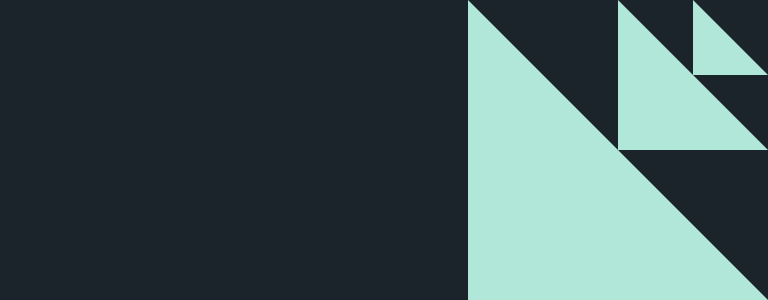
The Innovation Engine
The Innovation Engine podcast features interviews with best-selling authors and innovation experts from around the world. We focus on innovation in software and product engineering, corporate leadership, and emerging technologies.
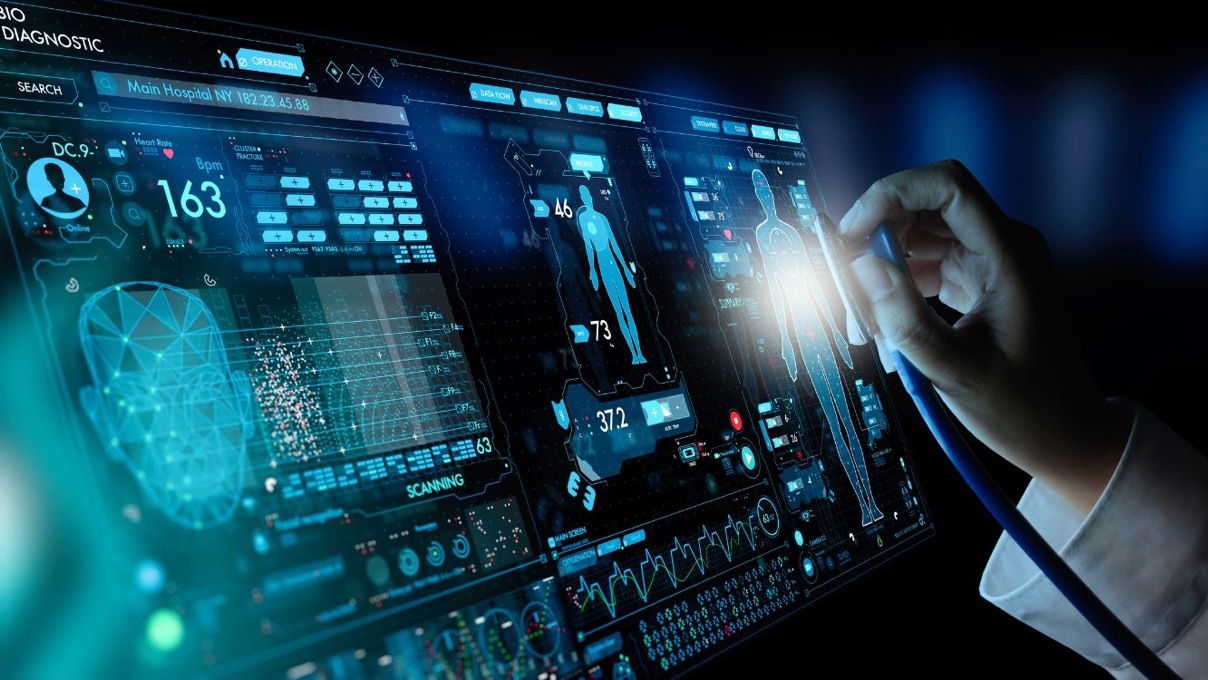
INNOVATION
Innovation in Healthcare: why the future has never been brighter
June 22, 2024Driving innovation in the healthcare space has traditionally been a challenge, to say the least. The good news? Many of the challenges that have stood in the way of unlocking healthcare innovation in the past are now fading into the background.
Read more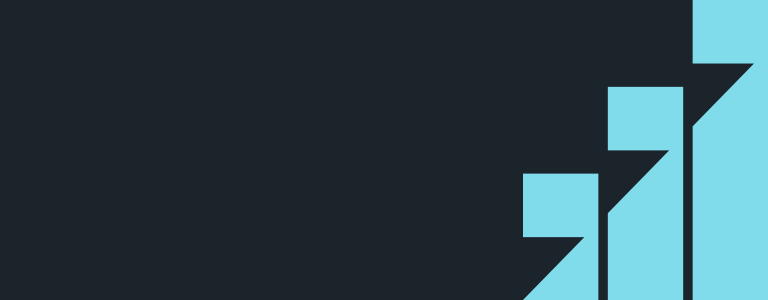
Stay in Touch
Keep your competitive edge – subscribe to our newsletter for updates on emerging software engineering, data and AI, and cloud technology trends.