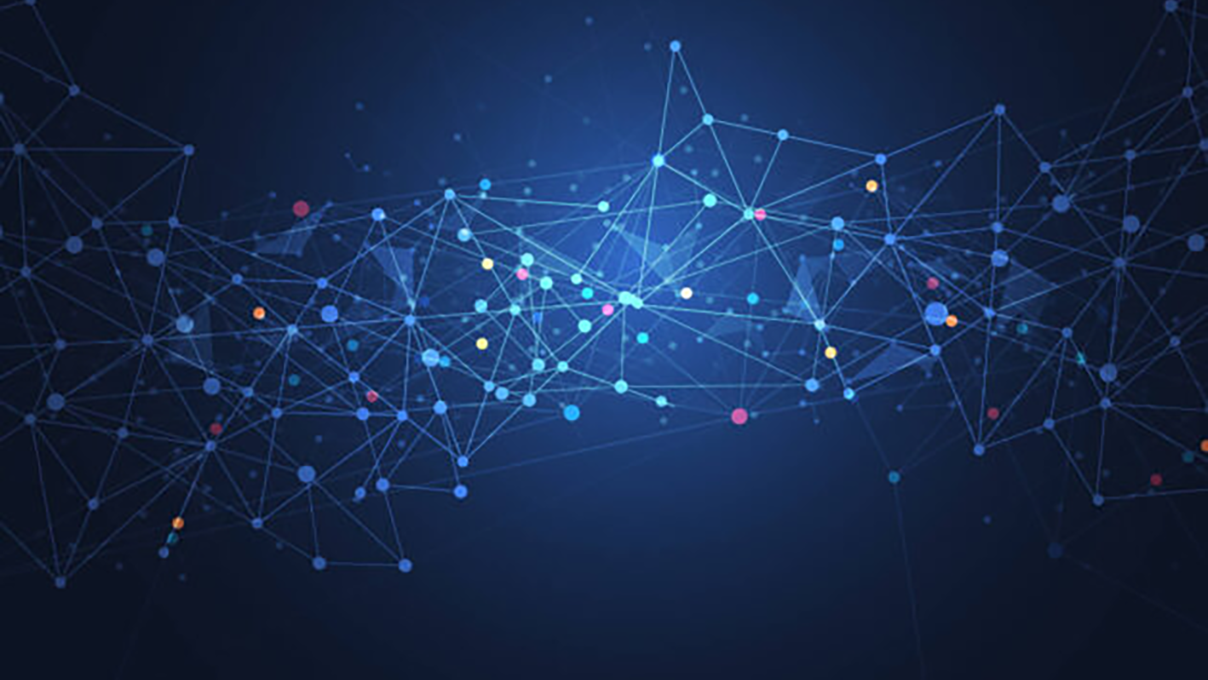
Building a Business Case to Launch Your Data Science Initiative
September 15, 2021
In this article, we examine how to make a business case for your data science initiatives. This includes discovering the “Whys” behind the initiatives and aligning them to key business strategies. We also present several data science use-cases. Arming yourself with this information will position you to get executive buy-in and the necessary budget to turn each data science venture into a big success. Most importantly, beyond executives buying-in, they will actually appreciate that someone has taken the time to explain exactly what data science can do to improve the health of the business.
Making Smarter Decisions and Smarter Products with Data Science
A semiconductor manufacturer, conducting every test on every chip design, began to reach its testing capacity. In an effort to avoid a large investment in new testing equipment, statistical analysis discovered 90% of the tests never failed. By identifying the test types falling into this category, the company eliminated some of those tests for certain chips and focused on the remaining 10%. This removed the need to buy new equipment to increase test capacity. Ultimately, the company was able to forego large capital expenses.This use-case provides an example of the benefits of data science. Others include applying data science on the farm to learn how to water more efficiently, based on machine learning applied to the analysis of infrared drone images of the soil. Or investors tapping into systems to use artificial intelligence to predict which pharmaceutical drugs to fund based on anonymized R&D reports that indicate which chemistries have a better chance of working with certain populations of people.
By leveraging artificial intelligence, machine learning, and other analytics technologies, initiatives like these enable companies to make smarter decisions and smarter products. While smarter decisions tend to improve operational efficiencies, smarter products deliver increased value to customers by generating information that leads to improved product performance or an enhanced user experience.
While the benefits of smarter decisions and smarter products are evident, business leaders still need to justify that a data science initiative will deliver sufficient ROI within a reasonable timeframe before making the investment. Perhaps you are analyzing a business case yourself or making a presentation to executives. Either way, before you start a data science effort, you need to find a way to internally sell your initiative.
First Determine the Whys
To prepare a presentation to get executive buy-in and the necessary budget for a data science initiative, start by defining the high-level reason—the Whys behind the initiative. For the decision or the product that you want to make smarter, what will the impact on the business be if you succeed? What are the consequences if your decision or product does not become smarter? Will there be ways to monetize the data, the inferences, or the APIs that create new revenue streams?Once you define the business need and document what a win looks like (with measurable goals), you can identify which decision(s) or which product(s) you need to make smarter, or perhaps a combination of the two. This also leads to identifying the correct data sources to fuel the decision(s) or product(s).
Identifying the data sources may also play a small role in making your business case; it helps identify potential costs (if you have to buy data from somewhere else) for a robust profitability analysis. Additionally, identifying data sources comes in handy once you develop the scope of the “data wrangling” when your team architects the solution.
Align Data Science to Business Objectives
Another key component when presenting your business case is to use a framework to focus on a specific area of the business that your data science effort pertains to. This shows executives how the data science activity aligns with key corporate strategies.One framework to consider comes from the book, The Discipline of Market Leaders by Michael Treacy and Fred Wiersema. Their model presents three dimensions—Operational Excellence, Customer Intimacy, and Product Leadership. Data science endeavors that map to one of these dimensions can focus on a specific range of business initiatives:
Business Dimension | Business Outcome | Measurable Impact |
---|---|---|
Operational Excellence |
|
|
Customer Intimacy |
|
|
Product Leadership |
|
|
For Customer Intimacy, Netflix provides a good example. As each subscriber watches a movie or a series, the program database uses data science algorithms to determine comparable content. Subscribers are then presented with suggestions of movies, series, and other programs that might interest them. And ultimately, they spend more time engaged with Netflix services, which increases loyalty and retention.
For Product Leadership, consider Intel, which bolstered its machine learning and AI capabilities in 2020 by purchasing data science startup Cnvrg.io. This acquisition demonstrates how the world leader in CPU chips expanded its product line to include development solutions that enable customers to rapidly build their own machine learning models.
Applying Metrics to Justify the Cost of Data Science
To demonstrate the ROI of a data science initiative, identify the specific business metrics that can be applied. Here are a few examples:- Accelerate order fulfillment times by 20%
- Lower customer churn by 10%
- Increase the average sale on new products by 25% and margins by 10%
- Save 20% per month on operational costs
- Avoid spending $350,000 on a mainframe lease by getting more from existing gear
- Raise the Net Promoter Score by 10 points
- Decrease net inventory by 5%
- Attract 25% more visitors per month to the company website.
By providing executives with projections such as these, it becomes easier to justify the cost of a data science initiative. They can see the added revenue that will be generated or the operational costs that will be reduced.
The Data Science Lifecycle
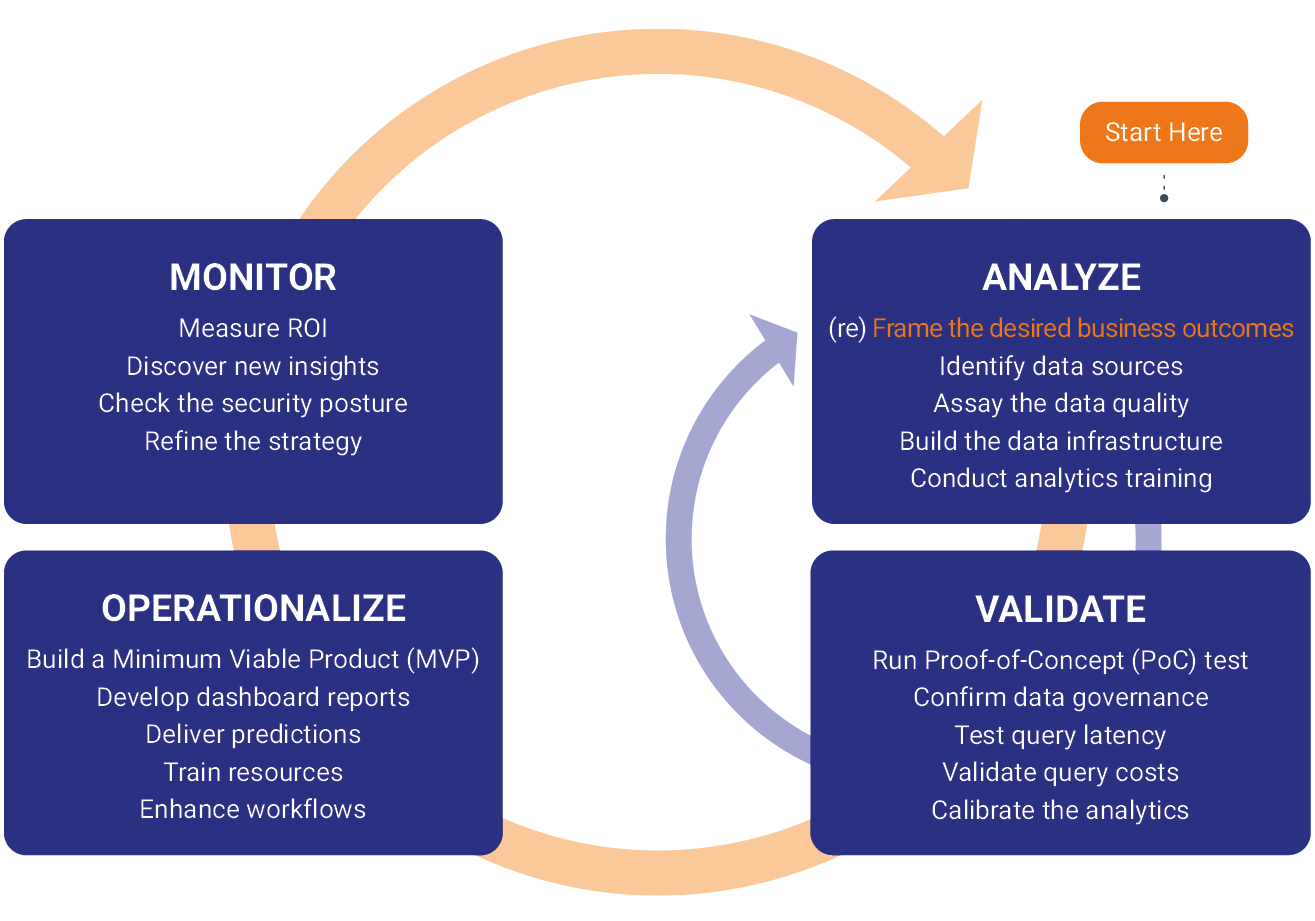
Data Science Use-Cases Across Industry Verticals
Data science can be applied to a variety of industry verticals as shown in these use-case examples:- Technology—A cybersecurity firm implemented data science to enable clients to improve their situational awareness of security threats. The solution tracks incidents while alerting clients when to raise their threat level ratings and change their security policies. The cybersecurity firm also applied artificial intelligence to track incidents against IP address clouds that cyber criminals use to spoof the identities of legitimate end-users. The clouds make it possible to change IP addresses, but there’s a limited set that threat actors can use. By watching clusters of IP addresses and clusters of events, clients can use the solution to determine if a user is a threat or a legitimate user. They can also scrape and match text to recognize when an attack mode is similar to another one that’s already been detected.
- Global Professional Services—A multinational professional services company uses data science to access intelligence to make optimal business decisions. They specifically wanted to maximize favorable currency exchanges when paying overseas employees, and by using machine learning algorithms, they took advantage of cycles in the exchange rates to adjust pay dates for different countries.
- Healthcare—A large medical product company wanted to make sure a data science initiative was working well, so they performed a check-up that involved a separate data science mission. Using deep machine learning tools for patient treatment determination, the company gets independent validation of its algorithms from an external regulatory agency. By using nearshore resources, they reduced data science engineering expenses immediately by 64%—with no impact on the product timeline, a net reduction in development costs, and lower regulatory certification costs.
- Real Estate—A commercial real estate company set out on a mission to develop a dashboard to deliver clear, accessible insights for agents selling property bundles to buyers. The agents needed clearer and more concise decision support so they could overcome problems with the original system. The company decreased asset inventory time and reduced the average time a property was for sale by 37% over a four-month project.
- Corporate Mergers—Following an acquisition, an executive management team required an audit to fully understand the acquired company’s data science decision support environment and how to apply it to the business strategies of the new unified organization. The incoming executive management team preserved the ROI on the previous management team’s $150K project and sustained the smart enterprise design after one 8-week project.
A Perpetual Cycle of Improvement
As you make the business case for your data science initiative by aligning its mission to business objectives and projected measurable results, you will be better positioned to win executive approval. And when you complete your mission to drive smarter decisions or smarter products, you will discover you have created a cycle of improvement that feeds itself. Each smarter decision has the potential to create smarter products. And each smarter product can enable smarter decisions. And thus the need for future data science activities!If you need help in making a business case for your data science initiative, contact us today.
About the author
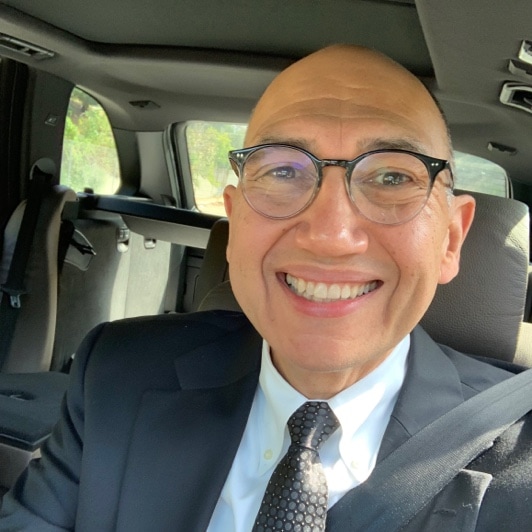
BY
SHARE