January 21, 2020
Challenges Of Artificial Intelligence
Artificial intelligence (AI) is poised to be one of the biggest things to hit the technology industry (and many other industries) in the coming years. But just because AI holds enormous potential does not mean it does not also have its challenges.
The challenges and possibilities are not small, which is why recognizing and working towards resolutions to problems can help further propel AI’s rapid growth. If this is your first time exploring the field, check out this high-level definition of artificial intelligence.
The Biggest Artificial Intelligence Challenges
Bias
Bias is one of the biggest challenges facing AI. Try as we might to generate data that is an absolute fact, there is inevitable bias when you explore the depths to which AI might be used. Forbes India explains the inherent bias in data: “An inherent problem with AI systems is that they are only as good—or as bad—as the data they are trained on. Bad data is often laced with racial, gender, communal or ethnic biases. Proprietary algorithms are used to determine who’s called for a job interview, who’s granted bail, or whose loan is sanctioned. If the bias lurking in the algorithms that make vital decisions goes unrecognized, it could lead to unethical and unfair consequences…In the future, such biases will probably be more accentuated, as many AI recruiting systems will continue to be trained using bad data. Hence, the need of the hour is to train these systems with unbiased data and develop algorithms that can be easily explained. Microsoft is developing a tool that can automatically identify bias in a series of AI algorithms.”
Computing Power
The tech industry has faced computing power challenges in the past. But the computing power to process massive data volumes to build an AI system that utilizes techniques like deep learning is unlike any other challenge previously faced by the tech industry. Obtaining and funding that level of computing power can be daunting for businesses, particularly startups.
Integrating AI
Seamlessly transitioning to AI is more complicated than adding plugins to a website or creating an Excel workbook enhanced by Visual Basic for Applications (VBA). One must ensure current programs are compatible with AI requirements, and that AI is implemented without stopping current output. The AI interface also needs to be set up in a way that infrastructure, data storage, and data input are considered, and that the output is not negatively affected. Additionally, once this is completed, all personnel must be trained on the new system.
Collecting and Utilizing Relevant Data
For an organization to successfully implement AI strategies and programs, it must have a base set of data and maintain a constant source of relevant data to ensure AI can be useful in the selected industry. Data can be collected on various applications with a multitude of formats such as text, audio, images, and videos. The wide range of platforms to collect this data adds to the challenges of artificial intelligence. In order to be successful, all this data must be integrated in a manner the AI can understand and transform into useful results.
Expertise
Because AI is an emerging technology, there are few who possess the skills or training necessary for AI development. Because this is a significant problem in the software development industry, many companies need to allocate additional budget towards AI development training, or the hiring of AI development specialists. McKinsey further explains the challenge of sourcing the skills necessary for artificial intelligence development: “With talent being one of the biggest challenges to AI, no matter how advanced a company’s digital program, it’s perhaps not surprising that companies are leaving no stone unturned when sourcing people and skills. Most commonly, respondents say their organizations are taking an ‘all of the above’ approach—hiring external talent, building capabilities in-house, and buying or licensing capabilities from large technology firms.”
Implementation Strategies
AI can transform almost every industry, but one of the major challenges is the lack of a clear implementation strategy. In order to be successful, a strategic approach needs to be established while implementing AI. This includes identifying areas that need improvement, setting objectives with clearly-defined benefits, and ensuring a continuous improvement process feedback loop. To compound the issue, managers need to a solid understanding of current AI technologies and their possibilities and limitations—as well as keeping up-to-date on the current challenges with AI. This will enable organizations to identify areas that can be improved by AI.
Legal Issues
One of the newest challenges of AI includes the recent legal concerns that organizations need to be wary of AI. If AI is collecting sensitive data, it might be in violation of state or federal laws, even if the information is not harmless by itself but sensitive when collected together. Even if the data collected by AI is not illegal, organizations need to be careful of any perceived impact that might negatively affect their organization. If the data is perceived by the public as violating their data privacy, the improvement for the organization might not be worth the potential public relations backlash.
Our teams recognize the issues and challenges of artificial intelligence and constantly improve how it is used in the digital products we create. Contact 3Pillar Global today to learn how we can help.
Free Whitepaper!
So You Want to Do Data Science: Now What?
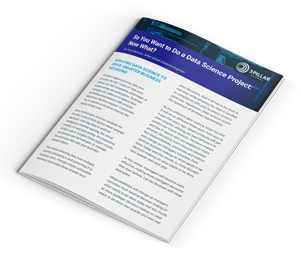
Download now to learn about:
- Applying Data Science to Make Smarter Decisions
- Getting Started
- Creating a High Level Framework
- Components of a Data Science Engine