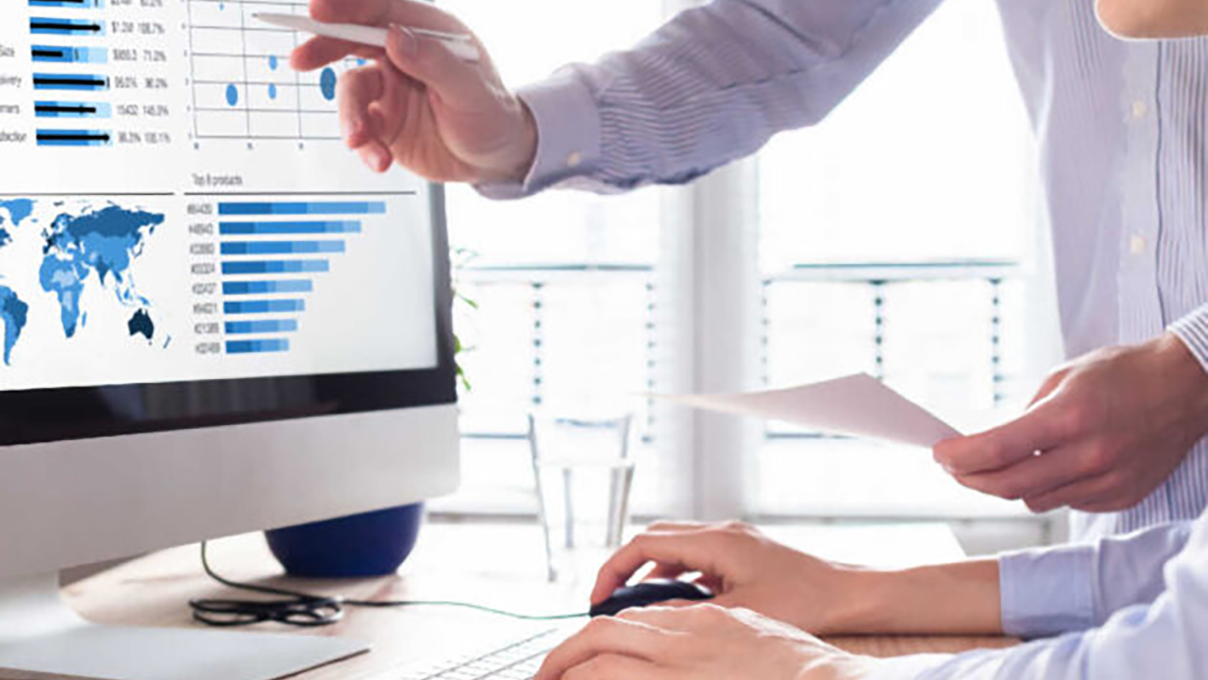
What Is Augmented Analytics?
Big Data has been called a strategic asset, a competitive advantage, and for a short while, “the new oil.” Today, the idea that data is valuable isn’t exactly news. However, many organizations don’t have a reliable way to transform raw data into action.
Today’s businesses have access to massive amounts of data, yet it’s becoming harder to manage all of those data points, identify what’s important, and chart a path toward making meaningful improvements. In this article, we explain augmented analytics, explore its business intelligence (BI) origins, and discuss the game-changing potential just over the horizon.
What Is Augmented Analytics and Why Does it Matter?
So, just what is augmented analytics?
Augmented analytics is a term coined by Gartner in 2017 that refers to the use of artificial intelligence (AI), natural language processing (NLP), and machine learning (ML) to enhance the value of data analytics. As it stands, most organizations understand that Big Data and advanced analytics are essential for doing business. Unfortunately, most businesses aren’t getting the full picture from their existing analytics solutions.
For example, your data might reveal a 15% decrease in revenue from last quarter. But that doesn’t tell you what that means for your business: Are market trends, supply chain issues, or poor ad performance to blame? Is it a quality issue? Or something else?
While analytics tools have become accessible to organizations of all sizes, humans are on their own when it comes to identifying the behind the metrics presented in their reports. Historically, extracting the insights that provide businesses with a competitive advantage requires the expertise only a skilled data scientist can provide.
Augmented analytics aims to help businesses get real value from their data by automating data management and insight generation. At the same time, augmented analytics makes it possible for non-technical users to generate reports and visualizations without writing an algorithm or relying on data scientists to do the heavy lifting.
The Evolution of Business Intelligence and Augmented Analytics
In order to understand augmented analytics and the potential it brings to the enterprise, it’s worth looking at the technology that brought us to this inflection point. Business intelligence (BI) is an umbrella term that describes the process of using historical data to make informed business decisions about future actions.
Though BI dates way back to the 1860s, BI tools have gone through some radical changes since the 1990s. Intelligence platforms have evolved from IT-generated functions into self-service tools designed for end-users. Today, we’re moving into the next stage in BI evolution: AI-driven augmented analytics. Let’s take a quick look at the evolution of BI platforms.
Traditional BI
Traditional BI tools are centralized systems that are owned and controlled by IT departments. Business users have few self-service options and little control over the data analysis process, which can create data silos within the company. This prevents co-workers from accessing insights that can help them improve individual and organization-wide performance.
That said, it’s important to note that traditional analytics tools aren’t necessarily outdated or irrelevant from a technological perspective. The main disadvantage here is, traditional intelligence tools prevent business users from accessing information that can help them make informed decisions.
Self-Service BI
Self-service BI was designed for the end-consumer and aims to make business intelligence accessible. Most analytics tools available today are self-service—think CRMs, website analytics, and marketing automation platforms. Typically, self-service tools are cloud-based and feature user-friendly interfaces and customizable reports that help users find insights themselves and draw their own conclusions.
Augmented Analytics
Over the past decade, business intelligence solutions have become more focused on gathering data from a wide range of sources and collecting that information into a single source of truth.
While sophisticated digital systems, algorithms, and cloud-based storage systems have largely replaced traditional solutions, many self-service BI tools still require manual preparation and human analysis.
Due to the widespread use of cloud-based applications, the rise of advanced analytics, and Internet of Things (IoT) technologies moving into the mainstream, organizations are generating more and more data. This makes it difficult to extract meaningful insights quickly enough to act on them without a little help from the machines. Luckily, AI, NLP, and ML capabilities have advanced to the point that many BI tasks can be automated.
Augmented analytics builds on the last generation of self-service BI tools and automatically correlates data and delivers real-time insights that guide business decisions. Augmented analytics platforms move away from dashboards and reports that define self-service platforms and rely on AI to present data through easy-to-understand visualizations.
What Is the Augmented Analytics Workflow?
The augmented analytics workflow refers to three key elements that must be in place for organizations to reap the benefits offered by augmented intelligence. Here’s a quick look at each of them:
- Data Preparation & Management. Augmented analytics applies AI and ML to activities such as data profiling, modeling, manipulation, cataloging, and harmonization—improving the process beyond what’s possible with stand-alone data preparation tools. Additionally, augmented analytics platforms are automating many activities associated with data management, ensuring a cleaner dataset that serves as the foundation for intelligent BI applications.
- Automated BI. Here, AI, NLP, and ML are embedded into BI platforms—allowing non-technical users to automatically identify relevant insights, create visualizations, and tell stories with data without writing algorithms or building models. The benefit here is that business users can identify untapped opportunities and relationships in seemingly unrelated variables across a wide range of data sets.
- Augmented Data Science and Machine Learning (DSML). Augmented DSML uses ML and AI applications to automate data science tasks, thus reducing the need for the specialized skills associated with generating, implementing, and managing an advanced analytics model. Instead, augmented analytics platforms democratize the process, opening DSML activities to citizen data scientists, developers, and analysts. These new capabilities aren’t meant to replace data scientists but instead give those skilled workers an opportunity to focus on high-value tasks.
What Is the Meaning of Augmented Analytics for Business?
The real value of augmented analytics lies in its ability to automate data analysis, identify patterns and relationships between data points, and uncover valuable insights hidden in untapped sources—all resulting in better business decisions and productivity gains. Here’s a quick look at some of the more specific ways that augmented analytics can benefit organizations:
- Ensures Data Accuracy At Scale. The more data you handle, the more opportunities there are for mistakes. It’s just a statistical fact. Even with trained data scientists on the case, manipulating multiple high-volume data sets could cause errors. By applying machine learning to data management processes, organizations can reduce instances of error, thus ensuring that all decisions are made based on accurate information.
- Automated Data Visualization. The data visualization solutions offered by augmented analytics allow users to view and analyze information, making it easy to identify a problem and its root cause and start moving toward a solution.
- Reduced Bias. In the context of augmented analytics, bias refers to human habits and routines that cause us to overlook sources that could contain valuable insights. AI-based platforms provide a more thorough data-capture process, ensuring that insights take all possibilities into account.
- Democratized BI. Augmented analytics democratize business intelligence by allowing non-technical users to access capabilities that previously required sophisticated data science skills. Instead, business users can simply enter natural language queries into a system to generate reports and visualizations to inform decision-making.
- Support for Data Scientists and IT. Since we’re discussing automation, it’s worth addressing the common misconception that AI will replace IT teams or data scientists. The reality is, augmented analytics allow both teams to focus on high-value tasks, as opposed to manual labor. IT teams have more time to dedicate to hardware and connectivity support, while data scientists can look for ways to capture even deeper insights.
How Businesses Can Take Advantage of Augmented Analytics
While maturity is a long way off, augmented analytics is poised to become the next must-have capability for businesses in all sectors. Early adopters have the potential to gain a significant competitive advantage compared to the firms that decide to hold off until augmented analytics go mainstream.
Right now, organizations should start taking steps toward creating an environment that allows them to get the most out of future investments. That might mean working to improve your team’s data literacy or fostering a company culture that sees innovation as an asset, not a threat.
[adinserter name=”Data Analytics CTA”]
Recent blog posts
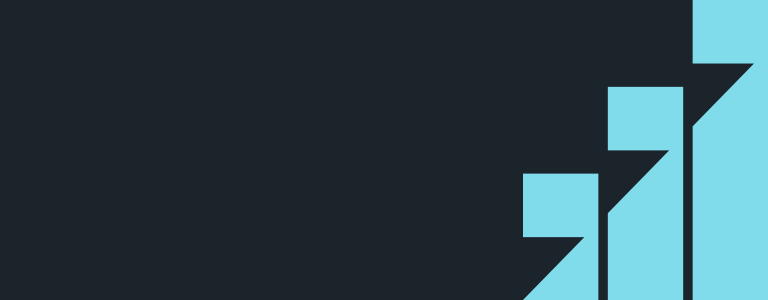
Stay in Touch
Keep your competitive edge – subscribe to our newsletter for updates on emerging software engineering, data and AI, and cloud technology trends.