Current Issues and Challenges in Big Data Analytics
In the last few installments in our data analytics series, we focused primarily on the game-changing, transformative, disruptive power of Big Data analytics. The flip side to the massive potential of Big Data analytics is that many challenges come into the mix.
A recent report from Dun & Bradstreet revealed that businesses have the most trouble with the following three areas: protecting data privacy (34%), ensuring data accuracy (26%), and processing & analyzing data (24%). Of course, these are far from the only Big Data challenges companies face.
In another report, this time from the Journal of Big Data, researchers reported on a whole range of issues related to Big Data’s inherent uncertainty alone. Additionally, Big Data and the analytic platforms, security solutions, and tools dedicated to managing this ecosystem present security risks, integration issues, and perhaps most importantly, the massive challenge of developing the culture that makes all of this stuff work.
In these next few sections, we’ll discuss some of the biggest hurdles organizations face in developing a Big Data strategy that delivers the results promised in the most optimistic industry reports.
Developing a Reliable Process to Capture, Analyze and Act on Data for Maximum Impact
One of the biggest Big Data challenges organizations face comes from implementing technology before determining a use-case. Essentially, they don’t know why they’re collecting all of this information, much less what to do with it.
As with any complex business strategy, it’s hard to know what tools to buy or where to focus your efforts without a strategy that includes a very specific set of milestones, goals, and problems to be solved. According to IDC, an estimated 35% of organizations have fully-deployed analytic systems in place, making it difficult for employees to put insights into action.
So before you do anything, what do you hope to accomplish with this initiative? Make sure internal stakeholders and potential vendors understand the broader business goals you hope to achieve. Data scientists and IT teams must work with their C-suite, sales, and marketing colleagues to develop a systematic process for finding, integrating, and interpreting insights.
A few things to consider:
How to Operationalize Data Across All Functions
As you consider your data integration strategy, keep a tight focus on all end-users, ensuring every solution aligns with the roles and behaviors of different stakeholders. This means that data scientists and the business users who will use these solutions need to collaborate on developing analytical models that deliver the desired business outcomes. End-users must clearly define what benefits they’re hoping to achieve and work with the data scientists to define which metrics best measure the impact on your business.
Additionally, you need to devise a plan that makes it easy for users to analyze insights so that they can make impactful decisions. How can you package data for reuse? What can you do to democratize data to support business goals at an individual level?
How to Scale Your Solutions
One of the biggest mistakes organizations make is failing to consider how their solution will scale. How will you handle your data as it grows in volume? What happens when the number of requests increases?
You will get the most value from your investment by creating a flexible solution that can evolve alongside your company. Using open source integration technologies will allow you to scale your solution or update your system with the latest innovations.
How to Ensure Stakeholders Receive Relevant, Accurate Information
In the Journal of Big Data report mentioned above, researchers found that as the volume, variety, and velocity of data increases, confidence in the analytics process drops, and it becomes harder to separate valuable information from irrelevant, inaccurate, or incomplete data. Analyzing massive datasets requires advanced analytic tools that can apply AI techniques like machine learning and natural language processing to weed out the noise and ensure fast, accurate results that support informed decision-making.
How to Control and Secure Data
You want to create a centralized asset management system that unifies all data across all connected systems. Creating a “single source of truth” isn’t just about pulling data in one place.
For one, you need to develop a system for preparing and transforming raw data. You also want to think about how a single source of data can be used to serve up multiple versions of the truth.
For example, the sales and accounting teams and the CFO all need to keep tabs on new deals but in different contexts—meaning, they review the same data using different reports. What policies and procedures need to be in place?
Ideally, you want to ensure you cover everything from governance and quality to security and determine what tools you need to make it all happen. Who needs to be involved in this process?
Will you be using tools that allow knowledge workers to run self-serve reports?
What About Data Privacy?
Protecting data privacy is an increasingly critical consideration. The General Data Protection Regulation (GDPR) and California Consumer Privacy Act (CCPA) are already in effect. Companies doing business with California or EU residents (which is just about anyone with a website) must now prove compliance with these regulations. They need a clear understanding of where data comes from, who has access, and how data flows through the system. Maintaining compliance within Big Data projects also means you need a solution that automatically traces data lineage, generates audit logs, and alerts the right people in instances where data falls out of compliance.
Data Validation
According to an Experian study, up to 75% of businesses believe their customer contact records contain inaccurate data. Data validation aims to ensure data sets are complete, properly-formatted, and deduplicated so that decisions are made based on accurate information.
Unfortunately, data validation is often a time-consuming process—particularly if validation is performed manually. Additionally, data may be outdated, siloed, or low-quality, which means that if organizations fail to address quality issues, all analytics activities are either ineffective or actively harmful to the business.
Data validation solutions include scripting and open source platforms. These require existing knowledge/coding experience or enterprise software, which can get expensive.
Managing Unstructured Data
Data management refers to the process of capturing, storing, organizing, and maintaining information collected from various data sets. The data sets can be either structured or unstructured and come from a wide range of sources that may include tweets, customer reviews, and Internet of Things (IoT) data.
Unstructured data presents an opportunity to collect rich insights that can create a complete picture of your customers and provide context for why sales are down or costs are going up.
The problem is, managing unstructured data at high volumes and high speeds means that you’re collecting a lot of great information but also a lot of noise that can obscure the insights that add the most value to your organization.
You can get ahead of Big Data issues by addressing the following:
- What data needs to be integrated?
- How many data silos need to be connected?
- What information are you hoping to gain?
Do You Need to Access Data in Real-Time?
Big Data can be analyzed using batch processing or in real-time, which brings us back to that point about defining a use-case. There’s a big difference in what you select for monitoring autonomous drones versus integrating customer data from multiple sources to create a 360 view of the customer. Will you use insights to predict outcomes? Identify opportunities? Simulate responses to changing environmental conditions, supply chain disruptions, or black swan events?
Cloud Computing
Many Big Data analytic tools are hosted in the cloud. On the surface, that makes a lot of sense. We’re used to SaaS tools with various reporting tools that tout being cloud-native as a selling point. And it is a selling point, especially when you’re talking about a project management app that enables remote work, a Google Doc you can edit from anywhere, or a service from your email service provider that automatically adds new subscribers and removes fake email addresses.
However, when you’re talking about Big Data, cloud computing becomes more of a liability than a business benefit. For one, most cloud solutions aren’t built to handle high-speed, high-volume data sets. That strain on the system can result in slow processing speeds, bottlenecks, and down-time—which not only prevents organizations from realizing the full potential of Big Data, but also puts their business and consumers at risk.
Cloud computing wasn’t designed for real-time data processing and data streaming, which means organizations miss out on insights that can move the needle on key business objectives. That lack of processing speed also makes it hard to detect security threats or safety issues (particularly in industrial applications where heavy machinery is connected to the web). Organizations wishing to use Big Data analytics to analyze and act on data in real-time need to look toward solutions like edge computing and automation to manage the heavy load and avoid some of the biggest data analytic risks.
Security
The sheer size of Big Data volumes presents some major security challenges, including data privacy issues, fake data generation, and the need for real-time security analytics. Without the right infrastructure, tracing data provenance becomes difficult when working with massive data sets. This makes it really challenging to identify the source of a data breach.
Here are a few areas to address as you consider Big Data security solutions:
- Remote storage. Off-site data management solutions from third-party vendors means data is out of your control. While some solutions offer comprehensive security, there is potential for a breach. You may want to consider applying your own cloud encryption key as a safeguard.
- Weak identity governance. Poor credential management leads to many problems, including complicated audit trails and slow breach detection. Big Data security requires granular access control (i.e., no one should have access to more information than their role requires).
- Human error. Implementation errors that happen while setting up new tools. Teams don’t always know how to secure every endpoint.
- IoT initiatives. IoT devices increase the potential threat surface by introducing more devices/endpoints to the network. These sensors and devices generate a ton of data and present several opportunities for hackers to gain access to the network.
Talent Shortages Present Many Big Data Issues
An EMC survey revealed 65% of businesses predict they’ll see a talent shortage happening within the next five years. Respondents cited a lack of existing data science skills or access to training as the biggest barriers to adoption.
Another survey from AtScale found that a lack of Big Data expertise was the top challenge. A Syncsort survey got even more specific; respondents said that the biggest challenge when creating a data lake was a lack of skilled employees.
Solutions like self-service analytics that automate report generation or predictive modeling present one possible solution to the skills gap by democratizing data analytics. In this case, business users like marketers, sales teams, and executives can generate actionable insights without enlisting the aid of a data scientist or an IT pro.
While that doesn’t address all of the talent issues in Big Data analytics, it does help organizations make better use of the data science experts they have. PwC recommends a few potential solutions:
- Hiring for skills versus degree requirements
- Investing in ongoing training programs that connect learning with on-the-job experience
- Partnering with multiple organizations and educational institutions to build a diverse candidate pool
- Developing mentorship programs
- Researching new ways to develop existing talent, like certificate programs, bootcamps, and MOOCs (Massive Online Open Courses).
Beyond a lack of data scientists and expert analysts, the rise of Big Data analytics, AI, machine learning, and the IoT means organizations face another set of Big Data analytics challenges: a changing definition of what types of skills are valuable in a changing workforce.
McKinsey’s AI, Automation & the Future of Work report advised organizations to prepare for changes currently underway. Humans will need to learn to work with machines by using AI algorithms and automation to augment human labor.
The firm stated that physical and manual labor skills are on the wane, but the need for soft skills like critical thinking, problem-solving, and creativity is becoming increasingly important. Additionally, the demand for workers who understand how to program, repair, and apply these new solutions is increasing.
Create a Data-Driven Culture
One of the biggest Big Data disadvantages has nothing to do with data lakes, security threats, or traffic jams to and from the cloud: it’s a people problem. According to the NewVantage Partners Big Data Executive Survey in 2018, over 98% of respondents stated that they were investing in a new corporate culture. Yet of that group, only about 32% reported success from those initiatives.
An article from the Harvard Business Review pointed out the “existential challenges” of adopting Big Data analytics tools. They stated that managers often don’t think about how Big Data might be used to improve performance—which is a significant problem if you’re using a mix of technologies like AI, IoT, robotic process automation, and real-time analytics.
Without the right culture, trying to both learn how to use these tools and how they apply to specific job functions is understandably overwhelming. To truly drive change, transformation needs to happen at every level. Leaders need to figure out how they will capture accurate data from all of the right places, extract meaningful insights, process that data efficiently, and make it easy enough for individuals throughout the organization to access information and put it to use.
Organizations need to develop procedures/training around the following:
- Interpreting data
- Storing data
- Cleaning data
- Processing/analyzing data
- Consumer trust/privacy
Beyond that basic roadmap, organizations need to focus on developing a collaborative environment in which everyone understands why they’re using Big Data analytics tools and how to apply them within the context of their role. Leaders must communicate the key benchmarks and explain to employees how data is improving processes and where things can be improved.
It’s also important to empower all employees with the tools they need to analyze and act on insights effectively. From there, you can integrate data science with the rest of the organization.
Preventing Big Data Challenges Starts with a Solid Strategy
From cybersecurity risks and quality concerns to integration and infrastructure, organizations face a long list of challenges on the road to Big Data transformation. Ultimately, though, the biggest issues tend to be people problems. Big Data along with AI, machine learning, and processing tools that enable real business transformation can’t do much if the culture can’t support them. Overcoming these challenges means developing a culture where everyone has access to Big Data and an understanding of how it connects to their roles and the big-picture objectives.
[adinserter name=”Data Analytics CTA”]
Recent blog posts
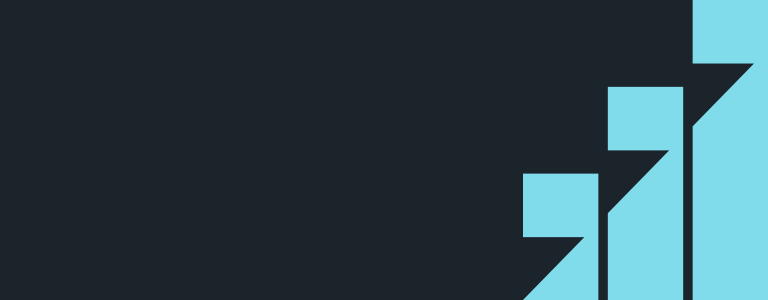
Stay in Touch
Keep your competitive edge – subscribe to our newsletter for updates on emerging software engineering, data and AI, and cloud technology trends.
Thank you for submitting the form