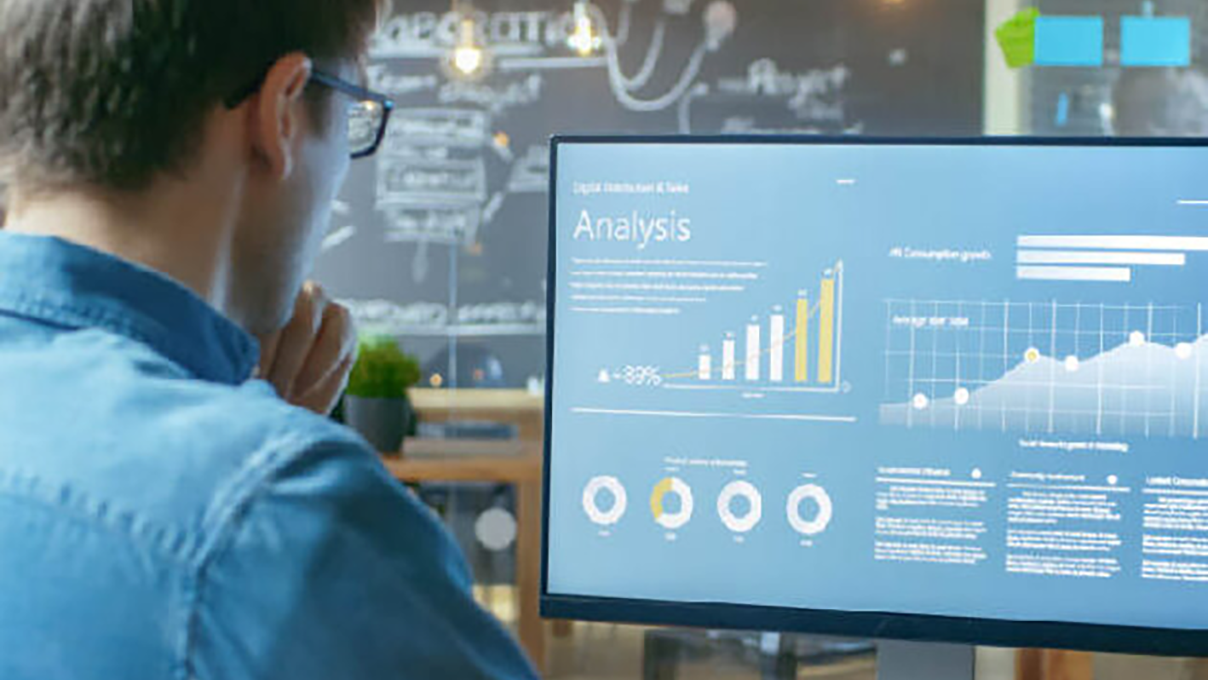
Creating Business Value with Data Mining and Predictive Analytics
According to a Splunk white paper titled The State of Dark Data, an estimated 55% of organizations’ data is dark, meaning it’s either inaccessible or unknown. At the same time, 71% of participating business leaders say they expect data to become more valuable within the next ten years, and nearly all said that they expect it to soon play a larger role in the decision-making process. What’s more, 76% of respondents believe that the company with the most data will come out on top.
“The more data, the better” isn’t the best way to think about data. Bigger datasets often create bigger problems, and without the right strategy and infrastructure in place, your most valuable asset remains out of reach. Businesses in every sector are looking toward two fundamental processes—data mining and predictive analytics—to combine and prepare disparate datasets, uncover new insights, and make game-changing decisions.
While data mining and predictive analytics represent different ways to extract information, both processes play a critical role in helping organizations make sense of their ever-expanding datasets and create measurable value. Below, we provide more insight into how both processes work.
The Difference Between Predictive Analytics and Data Mining
The best way to understand the difference between data mining and predictive analytics is that each term represents one half of a two-step process. Where data mining plays a passive role, predictive analytics aims to drive action.
Data mining uses software to capture, clean, and transform data, and uncovers patterns and relationships between disparate datasets. Done right, data mining gives users a clear picture of what’s going on.
Predictive analytics uses the information that surfaced during the data mining process to predict future outcomes, model different scenarios, and identify the best strategy for any given situation.
While the idea of gathering information and using those insights to develop a strategic plan is as old as time, predictive analytics and data mining allow this process to happen at speed and scale.
Here’s a bit more about the roles data mining and predictive analytics play in turning data into a high-value asset.
What Is Data Mining? Why Does it Matter?
Data mining identifies correlations, patterns, and anomalies in large datasets that humans can’t detect without some help from AI. It plays an essential role in uncovering the value in dark data and supports advanced technologies like AI, machine learning, and natural language processing (NLP).
Data mining also acts as a precursor to effective predictive analytics. It is used to provide businesses with two main benefits: describing historical data and uncovering meaningful patterns, rules, and relationships across disparate datasets that help predict future outcomes. Data mining allows businesses to learn more about their audiences, past trends, and current conditions.
Retailers might use data mining to learn more about their customers and break them into hyper-specific segments, allowing them to serve up tailored promotions to different audiences. In contrast, insurance companies can use insights to set rates and offer competitive products to their customers. Or educational institutions might use it to identify students at risk of dropping out, allowing for early intervention.
Data mining supports continuous data analysis, which, when combined with AI and machine learning capabilities, can be applied to automate decision-making, eliminating the need for human action. According to a Microstrategy report, 92% of respondents said they plan on deploying advanced analytics capabilities throughout their organizations, yet 38% say their existing solutions don’t support self-service, and 31% say they don’t have the skills needed to leverage tools.
As the Big Data landscape becomes ever-more complex, organizations must look toward new solutions. The more complex the dataset, the more cleansing, transforming, and organizing is required before it’s fit for analysis.
Those seeking real-time insights might look toward in-memory processing or edge computing to support immediate decision-making. At the same time, natural language processing and conversational analytics bring more context and understanding to end users.
Where Do Predictive Data Analytics Fit In?
Predictive analytics is a type of data analytics that uses Big Data, machine learning, predictive modeling, statistics, and AI to analyze existing data and predict future outcomes. Data mining is used to help organizations understand a situation. Predictive analytics takes on a more active role, enabling users to anticipate outcomes and develop proactive strategies for a wide range of future scenarios.
While predictive analytics doesn’t technically predict the future, it analyzes the relationships between data points and assigns risk scores to certain conditions based on historical data.
Done right, predictive analytics helps brands act on promising opportunities and avoid crises on the horizon.
For example, predictive analytics is often used by sales people to score leads based on propensity to buy. In contrast, customer success teams use this technology to prevent churn and maximize customer lifetime value.
Manufacturers and fleet managers can use predictive modeling to predict machine wear and tear, preventing costly repairs and downtime and allowing organizations to schedule service as needed. While Big Data analytics and predictive modeling add significant value to organizations, leveraging those benefits will require training and ongoing support.
In the Splunk report mentioned above, there’s a gap between the perceived need for dark data and the skills and resources to mine and analyze said data. Researchers found that 83% of respondents believe workers who continue to rely on others to explain Big Data insights will soon fall behind in their careers.
Yet, 69% said they themselves are content to maintain the status quo, even if that means they never get another promotion. What’s more, about half of that group says they’re “too old” to learn new data skills.
Though we can’t speak for the anonymous respondents, it’s easy to conclude that workers are intimidated by Big Data and don’t feel confident that they can evolve alongside changing job requirements. That said, Big Data predictive analytics tools are evolving to cater to a broader range of workers. We increasingly see analytics platforms that suggest actions based on the predictions made, along with explanations for how the AI came up with the recommendations.
Unlike traditional analytics tools that rely on statistics, predictive analytics platforms use technologies like AI, machine learning, and predictive modeling to analyze available data and forecast potential outcomes. Modern tools even make data analytics accessible for the workers who previously felt like Big Data was out of their depth.
We now see platforms that provide AI-driven explanations and visualizations, and which allow users to enter custom queries in plain language, much like a Google search. Still, as McKinsey researchers pointed out in a 2018 paper, creating business value from Big Data analytics is more about empowering workers and embedding analytics into organizational culture and day-to-day tasks, and less about the latest tools.
Predictive Analytics and Data Mining Work Together to Bring Value to the Business
Data mining and predictive analytics are two separate, yet closely linked processes, each with an essential role in turning data into real value. Without good data, predictive analytics offers little in the way of business value. After all, making business decisions based on inaccurate information can cause damage to your reputation, your customer base, and your bottom line.
Data mining allows you to filter out the noise in your datasets, identify anomalies and duplicate records, and find and present relevant information to support informed decision-making. Still, without AI-driven analytics in the mix, humans must form their own conclusions—and, let’s face it, we can’t compete with the bots!
[adinserter name=”Data Analytics CTA”]
Recent blog posts
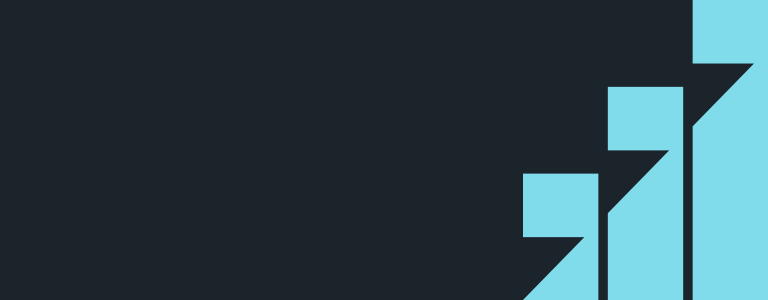
Stay in Touch
Keep your competitive edge – subscribe to our newsletter for updates on emerging software engineering, data and AI, and cloud technology trends.