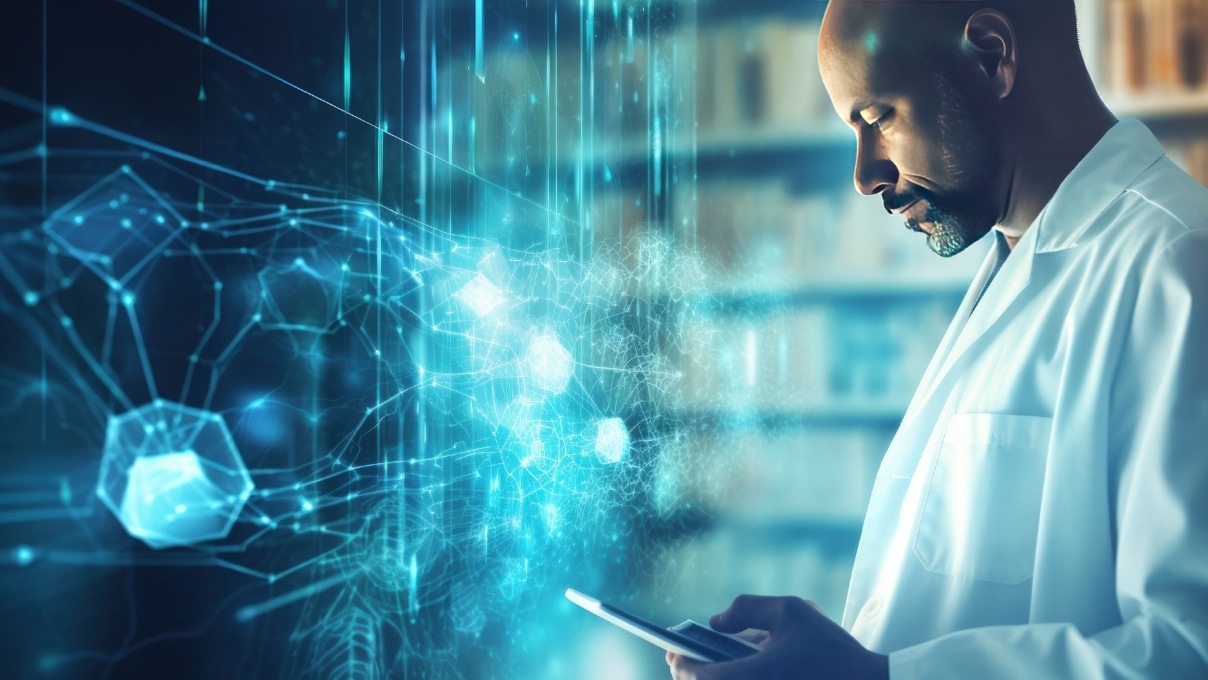
How Providers & Health Systems Are Leveraging AI to Minimize Claim Denials
June 11, 2024
Earlier this year, CMS announced “concern” over use of AI algorithms in denying claims coverage. Specifically, they announced a rule providing multiple guardrails for Medicare Advantage payers: AI can’t make decisions, must be compliant with larger agency requirements, and decisions must be made on individual patient history, not broad datasets.
While concerns about bias and discrimination are warranted, the CMS rule fails to address the core problem underlying claims denials. It’s not that AI algorithms are in play. It’s that the denial rationale is hidden from providers and the patients they serve.
Rather than view AI, ML, LLM, and algorithms as the enemy, providers should seize an exciting new opportunity: to recreate health plans’ internal rules engines to more efficiently submit clean claims. This can not only improve back-office and mid-cycle efficiency, but stave off the concerns of bias and discrimination that CMS wants to prevent.
Read on to learn more about how to create this kind of AI-powered engine and the benefits it offers your entire health system, read on.
How did we get here?
Why is it so difficult to submit clean claims? Why is this the accepted norm? The reason is a natural conflict existing between providers and health plans: every claim submitted is revenue for the provider, and every claim paid is a cost for the health plan. This natural tension has led to a laundry list of challenges. For starters, health plan interfaces are a mess. Worse, they vary significantly based on the health plan in question, which requires institutional knowledge to navigate the path to a clean claim: different diagnostic codes, prior auths, eligibility requirements, and a bunch of stipulations. Granted, all of these are documented in the hundreds of pages of PDFs from the health plan. But clinicians and coders aren’t reading the fine print on every page across all the plans you work with. Plus, these PDFs aren’t incorporated into your RCM system. So even if you were using that data, you’re going back to that document every time you submit a claim—this is slow and painstaking to say the least. The reality is that office staff keep all this knowledge in their head and input the data manually. While that can work to some degree, you’re opening yourself up to human error—and putting yourself into a bind when one of those back-office staff members decides to retire.So what’s the solution?
Providers have an opportunity to leverage GenAI to actually reverse-engineer health plans’ algorithms, using those insights to streamline claim submissions across the entire organization. We’ll break this down into three stages: back-office, mid-cycle, and front-office.Back-office: recreate the health plan algorithm
Providers and their vendors all struggle to submit claims in a way that maximizes acceptance and reimbursement rates. Since health plans conceal their denial rationale, this is easier said than done. But here’s the good news: you have all the information you need to recreate each health plan’s unique rules engines. The problem is this data is scattered across historical acceptance/denial data, documentation, and institutional knowledge. It’s far too complex for a human to capture, collate, and analyze. That’s where GenAI and ML come in:- Use AI to extract historical data from past claims and tease out the appropriate rules—any mistakes are incorporated into the rules-based model and never made again
- Analyze your documentation: although clearing houses provide modern API documentation, these PDFs (hundreds of pages long) aren’t health plan-specific. Use AI to ingest publicly available call data and incorporate it into your rules engine.
- Leverage institutional knowledge by deploying configurable rules engines and subsequent robotic process automation (RPA) to capture and automate what your staff already knows—before they retire
Mid-cycle: provide real-time coding assistance
Once you recreate the rules engine on the back end, you can pull those insights forward to the mid-cycle. This enables clinicians to retrieve the information necessary to properly code claims at the point of need—not weeks or months later when a denial comes through. Naturally, this not only speeds up the process, it creates a more seamless and pleasant work experience for everyone within your organization. This can occur in three dimensions (in increasing order of good to best):- Create a rules engine to flag potential documentation issues. After providers document and generate claims, an RPA-based application and workflow prompts potential billing issues, suggesting ways to fix it.
- Autocomplete and co-pilot billing. The RCM system pulls historical data from the EMR and slots them into relevant fields.
- Ambient listening + real-time questions. These systems enable providers and their vendors to ask additional questions relevant to billing in real time, as well as give patients and doctors information about next steps, including real-time information about approximate costs.
Front office: Engage patients upfront
Once you’ve recreated health plan algorithms and built systems to flag potential issues in the mid-cycle, the natural next step is to bring those capabilities to the front office. These enable front-office staff to fix a number of the issues they face:- Digital check-in. Build a quality UI into your RCM system to engage patients upfront. The more you can customize your workflows to get the exact information you want, the better the overall patient experience will be.
- Improved eligibility data interpretation. Move beyond simply verifying active coverage and go deeper to retrieve data regarding secondary coverage, collect deductibles at the point of sale, and verify whether the current visitor is covered.
- Price transparency & POS collection. When coding happens in real-time, you’re able to have greater price transparency with your patients, and even collect payment at the POS. Problem is: most POS systems were built for copay plans, but most people now have deductibles. As such, calculating costs at the POS is more complicated than it’s been historically. An increased amount of automation and data is key to actually achieving this at the POS.
Final thoughts on reverse-engineering payer rules to maximize clean claims
What we’re talking about isn’t theoretical. We’ve actually implemented it and driven significant success for providers. In one case, we built this approach to help a health provider achieve the following results:- Decreased claims revenue cycle time from 6 weeks to 2 weeks
- Increased auto-adjudication of claims by 67 percent and improved claims processing accuracy to 97 percent
- Reduced time to onboard and credential new providers by 60 percent, and doubled claims processing capacity with existing staff
- Enabled payer to ramp up from processing a few thousands claims per day to 75K+ claims per day as customer base scaled
About the Author
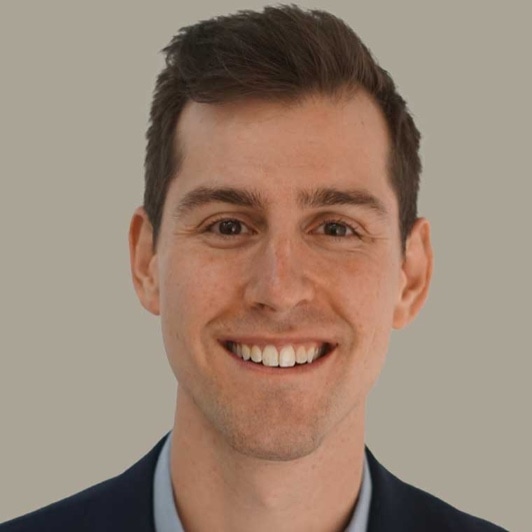
BY
SHARE
Recent blog posts
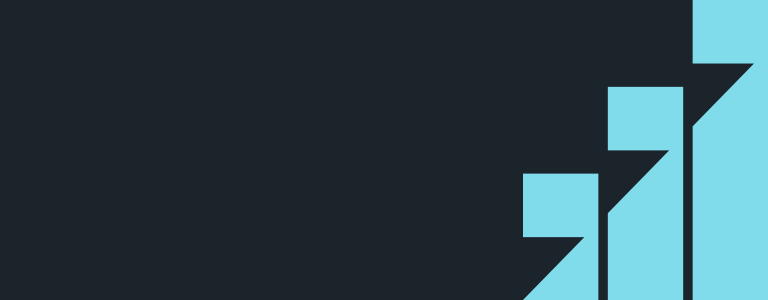
Stay in Touch
Keep your competitive edge – subscribe to our newsletter for updates on emerging software engineering, data and AI, and cloud technology trends.