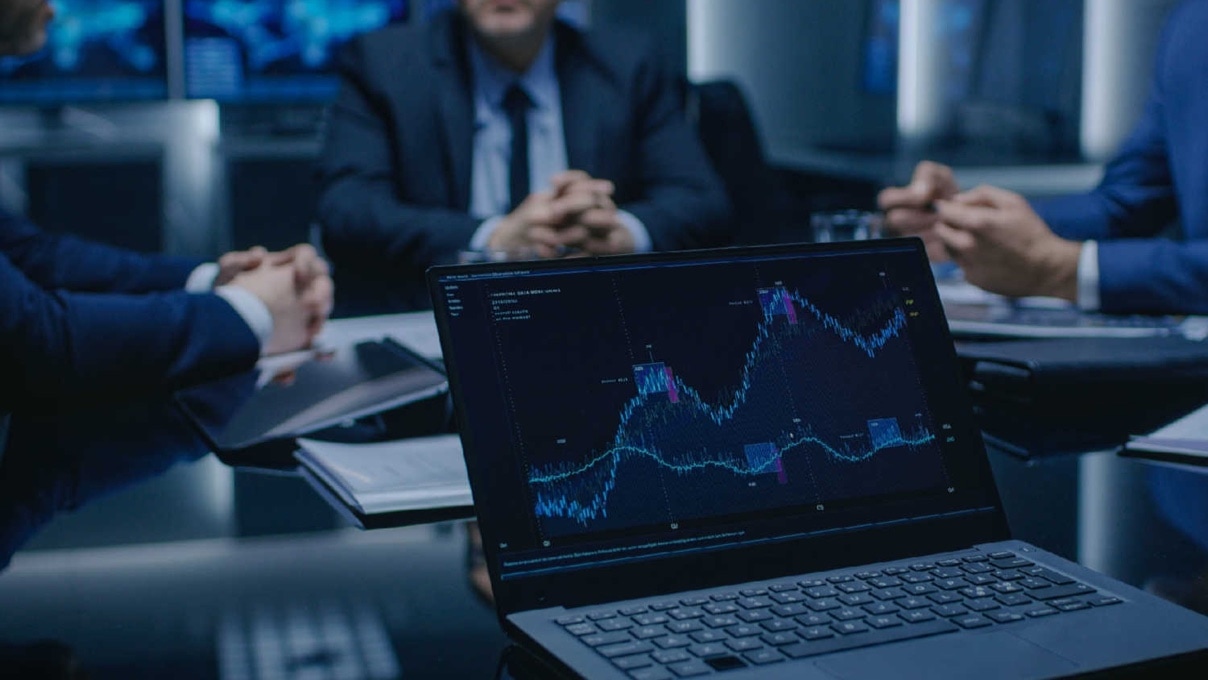
Best Practices for Managing Big Data Analytics Initiatives
Updated: April 18, 2022
From machine learning to artificial intelligence (AI), big data seems to only be getting bigger over time.
According to Sigma Computing, the big data industry is projected to be worth a staggering $77 billion by 2023. And while many organizations believe they’re in that data-driven group, few have truly mastered the art of leveraging big data to gain a competitive edge, as only 14% of companies make their data accessible to employees.
In this article, we’ll cover some of the best practices for managing big data, as well as how to avoid the chaos that comes with poor planning.
Define Your Big Data Strategy
Many enterprises have mastered “small data.” When dealing with smaller data sets, it’s relatively easy to extract meaningful insights and identify trends, patterns, and opportunities. Big data, on the other hand, is largely unstructured and random until you’re able to organize it. Without organization of big data, actionable insights are tough to come by, and uncovering them is nearly impossible.
To set the stage for managing big data successfully, your data strategy must become your business strategy.
Start by identifying high-level business objectives and potential use cases.
- What data will you need to achieve those goals?
- What stakeholders need to be involved?
- What are their roles and responsibilities within the context of this project?
Avoid the impulse to collect as much data as possible. Focusing on capturing data before you have a strategy in place puts brands at risk. You can’t make data-driven business decisions when you can’t verify whether that data can be trusted.
Identify Useful Data Islands and Eliminate Silos
As you begin to implement your strategy, identify which data “islands” contain valuable insights that could help you streamline your processes or deliver the perfect solution to your customers.
Then incorporate those data sources into a “single source of truth.”
According to Jeanne Ross, director of MIT Sloan School’s Center for Information Systems Research, a unified view is more about alignment than accuracy. Essentially, establishing alignment early in the process allows organizations to develop a shared language for discussing strategic initiatives and defining how to measure success.
Make sure you—and your team—understand the difference between data and information. While it may sound like an issue of semantics, the distinction is an important one. Data is information in its rawest form. Not all data can be extracted and turned into action, but information gives data context. You want to avoid incorporating data islands that don’t add value.
Managing Big Data Analytics Projects Is a Collaborative Effort
When it comes to how to manage big data, the process is definitely a team effort.
Data science, IT, and other stakeholders need to align on goals, which means that organizations need to create an environment where collaboration and agile practices are baked into the culture.
However, culture is one of the hardest parts of taking on a large-scale transformation. This holds true whether you’re embracing the Internet of Things (IoT), migrating to microservices, or developing big data analytics initiatives that set your brand up for success.
Make Data Accessible
In most businesses, data is severely underutilized, often sitting on a database that’s rarely looked at. Getting the necessary insights means enlisting the help of experts who can decipher and convert complex data into actionable insights.
Given the complexities associated with managing big data, there’s a massive shortage of professionals with data science and IT skills. These professionals are needed to help businesses make the most out of their growing data sets.
To combat this, organizations need to make it easy for employees to find the information they need. What’s more, those employees need the relevant context to fully understand the data and use it to make informed decisions.
Employees need ongoing training to ensure they’re finding and leveraging the right information. Employers should also consider assembling a tech stack that makes life easier on their employees. Additionally, leaders should provide secure access to large, high-quality data sets to encourage experimentation and discovery among employees outside of the IT department.
Fix Data-Access Issues
When data lives in isolated systems, it’s impossible to use siloed information to improve decision-making, streamline operations, or gain a big-picture assessment of what’s happening inside an organization.
According to research from Hosting Tribunal, Fortune 1000 companies can gain more than $65 million additional net income when they increase their data accessibility by 10%.
So aim to break down boundaries between data science and the rest of the organization—by creating centers of excellence for sharing knowledge, proof of concept results, and data sets that cross department lines.
Offer Just-in-Time Training & Ongoing Skills Development
Prioritizing data literacy initiatives yields a variety of benefits. Tableau reports that nearly 80% of employees are more likely to stay at a company that offers data skilling programs.
To help everyone on your team make sense of big data and maintain privacy and security standards, you can implement the following solutions:
- Help others begin to use data for the first time in their job
- Provide education and mentoring to bring less-technical employees up to speed
- Hire based on actual skills
- Adjust company culture so that staff will justify decisions they make based on data
Provide Tools for Helping the Entire Organization Work with Data
Teams need data analytics tools that simplify data prep and analytic tasks and save time. That’s why it’s critical to offer solutions that allow users to reuse reports and templates.
It’s also important that users can pull from connected data sources to create predictive models and run ad-hoc queries on-demand. AI, natural language processing, machine learning capabilities, and automation streamline processes and offer insights impossible for humans to detect on their own.
Many tools also help explain relationships between data points as well as offer visualizations that make it easy for non-technical professionals to arrive at conclusions and make informed decisions.
Data Governance
This might not be the most exciting part of the data analytics process and best practices, but it will protect your brand from compliance breaches and audits. As more people in an organization access data sets, build models, and run queries, you need to make sure you have the right data governance practices to maintain the integrity of your data and models. Governance is critical as it allows organizations to set rules, permissions, and policies to protect your data via automated workflows.
A few areas to focus on:
- Data catalogs and dictionaries—A centralized dictionary helps organizations categorize, tag, and organize data for easy access. It also helps users identify metadata from existing data sets and ensures everyone is on the same page when it comes to business terms, descriptions, and conditions. While this may seem like a small thing, the common language eliminates confusion, encourages collaboration, and maintains consistency across various platforms and databases.
- Data lineage—Data lineage provides an audit trail for data, allowing businesses to track data movements, identify relationships to other data, and reveal which users and tools have access to information and why. Data lineage also plays a critical role in maintaining GDPR, HIPAA, and CCPA compliance, especially as data sets continue to grow exponentially. Additionally, lineage plays an important role in AI applications, particularly in areas like deep learning, machine learning, and neural networks. Data lineage records can help systems learn complex patterns based on human interaction data, providing a faster path to full automation.
- Model management—Consider using a tool that automates model monitoring processes and can send alerts when a model begins to degrade. You want to avoid any potential scenario where you make decisions on a model that no longer works—especially if you use modeling to determine something like patient outcomes.
Keep Evolving Your Data Analytics Best Practices and Processes
Big data continues to grow exponentially and isn’t likely to slow down anytime soon. In fact, companies that harness big data’s full power are projected to increase their operating margins by up to 60%.
With that being said, data strategies should be treated as living documents, while cultures should show continuous improvement.
Again, you want to make sure you stay focused on those same goals you defined at the start of this process. Ask yourself (and your team) the following questions to benchmark your progress:
- Which metrics represent success?
- How are you tracking progress?
- What can you do to evolve this strategy?
- Are there opportunities to add new data sets to existing strategies to provide even richer insights?
- Could your team be more efficient or productive?
Over time, you will begin to introduce new data hubs, automate more processes, and address the biggest problems facing your business and your industry. You might also adjust your reporting process, add new metrics, and ditch data sets deemed ineffective.
[adinserter name=”Data Analytics CTA”]
Recent blog posts
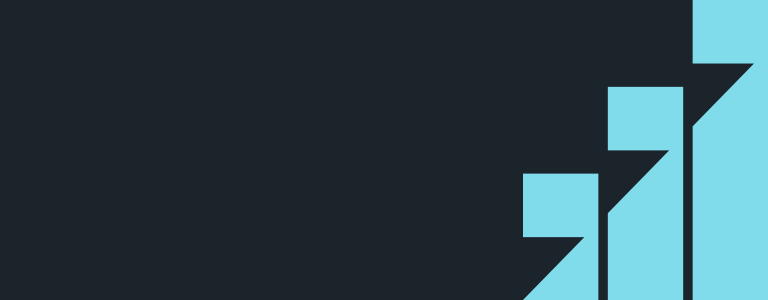
Stay in Touch
Keep your competitive edge – subscribe to our newsletter for updates on emerging software engineering, data and AI, and cloud technology trends.