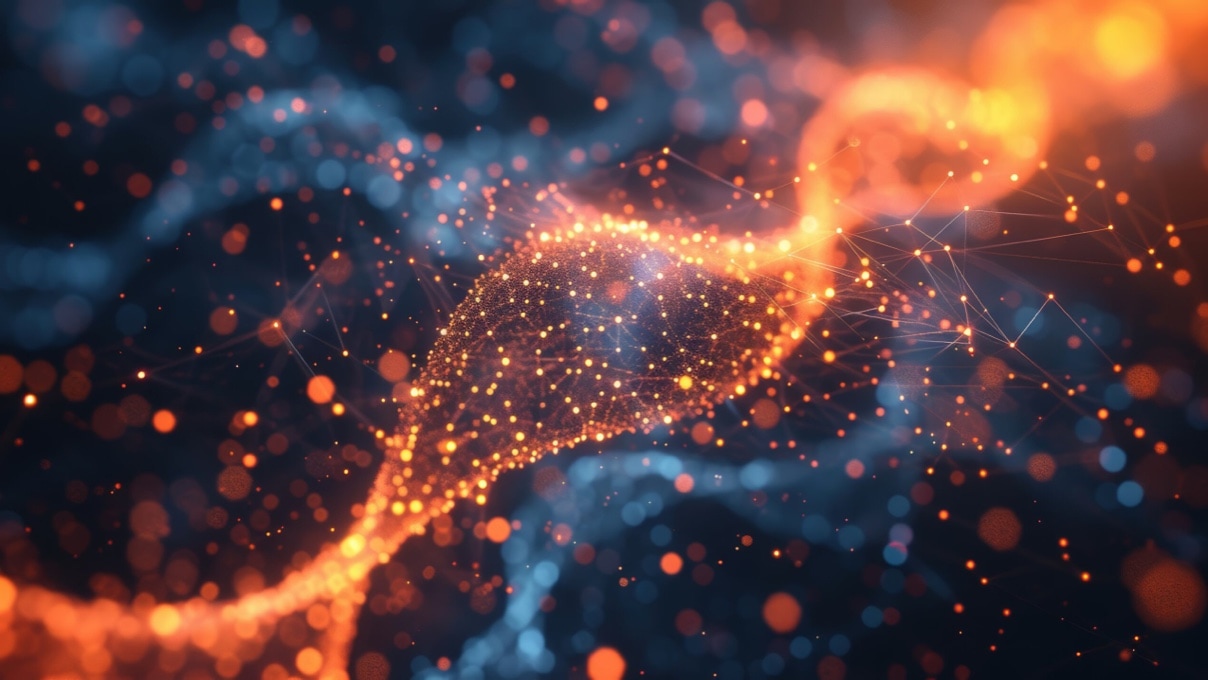
Why the Marriage of LLMs & LAMs is the Missing Link in AI Utility
The impending intersection of Large Action Models (LAMs) with Large Language Models (LLMs) will trigger a leap forward in the applications of AI in business settings, blending advanced comprehension with intuitive action. This intersection, which will be upon us before we know it, will be the missing link that turns AI from being a novelty/curiosity into a true utility that businesses can’t live without.
This fusion of LAMs and LLMs is set to redefine business models, enhance customer experiences, and introduce innovative products and services. The core of this transformation lies in leveraging AI’s dual capabilities: understanding human language through LLMs like ChatGPT and executing tasks via LAMs like one that Apple is signaling work on and the Rabbit r1.
What are LAMs, and How are They Different from LLMs?
LLMs are well known by now. ChatGPT, Gemini, Claude, Perplexity, and the like have entered the public consciousness for good. LAMs aren’t nearly as well known, but that’s going to change in a big way in the very near future as more people get their hands on hardware that’s AI-enabled or, in other words, are LAMs.
To understand where LAMs may be going, a number of Apple researchers recently published a research paper exploring how to combine the power of LLMs with LAMs in the Apple operating context on Apple devices. The whitepaper foreshadows significantly improved UX of LLM performance on smartphones.
The Rabbit r1, which drove $10 million in pre-orders before anyone could even lay a finger on it, is shipping to its first cohort of customers this week. And the growth rate of AI PCs is expected to skyrocket over the next few years, with AI PCs making up 60% of all PC sales by 2027.
So, what are LAMs, and what’s the difference between them and LLMs?
LLMs require prompting, which limits their utility. Great as ChatGPT is, you still have to tell it what to do. And the results, as you’ve likely experienced, are often mixed. I liken LLMs at this stage of their development to talented interns or junior level staff.
By the time you’ve crafted the perfect custom GPT to get exactly what you want, or gone back and forth with ChatGPT until you get a suitable response to your prompt, you often could’ve just done the task yourself. You can see the vast potential for the technology and the glimmers of hope in LLMs. The follow-through, however, leaves something to be desired. Enter LAMs.
Unlike LLMs, which are trained on large data sets, LAMs learn by observing or training on the world around them and then execute tasks based on what they’ve learned. LAMs are not AI models but carry out actions in a sequence that carry out complex assignments or workflows. Let’s look at just a few real-world examples where the combination of LLMs and LAMs could prove to be revolutionary.
Real-World Use Case #1: Next-Level Robotic Process Automation and Business Process Management
I spent nearly a decade in the early 2000s building technology products in the mortgage lending industry. During that time, I became quite familiar with the rules and logic that companies use to determine if a potential home-buyer would be approved for a loan. Pre-existing business rules include a mix of considerations. They include a would-be buyer’s credit score, employment history, liquidity, how large a down payment they could put down, etc.
Even today, more than a decade later, the home-buying process is still one that involves a high degree of manual input, review, and approvals. Listing agents, buyers’ agents, mortgage officers, title officers, inspectors…there’s an awful lot of red tape involved in the entire home-buying process, much of which could be automated away by using LAMs + LLMs.
What if mortgage officers had vast data sets on prospective buyer history to pull from rather than relying on historical artifacts like credit scores? And if we’re being honest, how much of the closing process could be digitized and automated?
The traditional credit score and predictive machine learning are still valuable. But a single number and a predictive classification are no longer in the driver seat. These are just contributing factors to the powerful reasoning LLM agent.
While the LLM agent is making a plan and reasoning a decision, the LAM component will provide the operating context in the mortgage process that takes the automated transaction step.
The entire home-buying process is just one example of a relatively antiquated set of business processes that could be revolutionized with AI.
Real-World Use Case #2: A New Frontier of “Pair Programming”
Pair programming has been a staple of the software development industry for decades. The idea itself is simple: two engineers sit together to write code, with one developer writing the actual code and the other developer reviewing the code for bugs or defects while generally acting as a sounding board. In the “old” model, the engineers would frequently change roles.
I see a day coming soon where, rather than having a human pair programmer, AI bots or agents will be the ones writing code while humans increasingly play the role of quality control. This will go even further than the low-code/no-code application platforms that have made it dead simple for people with limited engineering knowledge to write functional business applications.
There was a time not long ago when there was a common rallying cry: “Every company is now a tech company.” It won’t be long before that rallying cry becomes instead “Every company is now an AI company.”
The Impact and Future Outlook of LAMs + LLMs
The fusion of LAMs and LLMs represents a pivotal development in the journey towards more sophisticated and utility-focused AI agents in business contexts. As these technologies continue to evolve and integrate, they promise to redefine the landscape of business innovation, offering new pathways for growth, efficiency, and unparalleled customer experiences. The exploration of this intersection is not just about technological advancement; it’s about opening doors to new possibilities that were previously beyond reach.
To put it in perspective, today, we view the Internet as indispensable in the workplace. That wasn’t the case in the early days of the Internet, when dial-up modems and Netscape and AOL reigned supreme. I’d equate where we are now with AI to those early days of the Internet. The coming combination of LAMs and LLMs will be a powerful blend that shapes the future of solid applications of AI and provides foundational building blocks for companies to utilize AI to develop innovative new products and services.
About the author
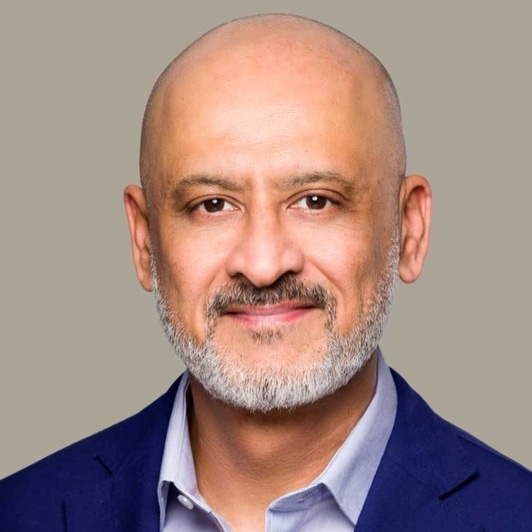
Recent blog posts
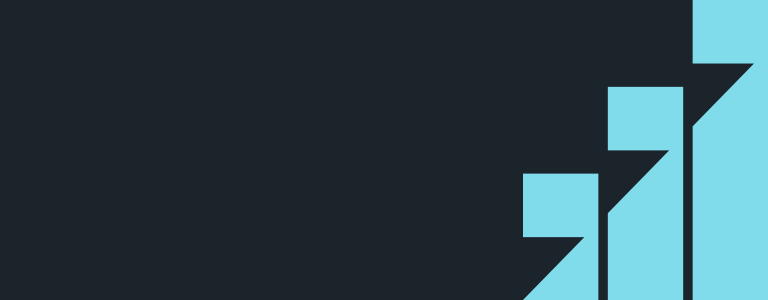
Stay in Touch
Keep your competitive edge – subscribe to our newsletter for updates on emerging software engineering, data and AI, and cloud technology trends.